From an operational standpoint, the role of robotics and automation in battery swapping is pivotal. Automated guided mechanisms handle each module, reducing labor costs and minimizing human error.
Automation’s Future in Sustainable Transportation
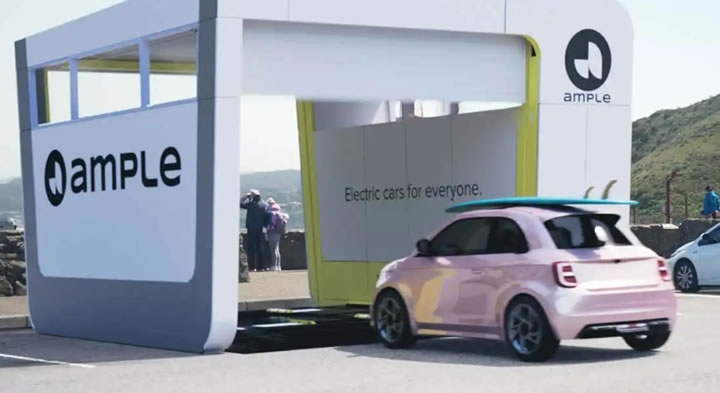
Automation’s Future in Sustainable Transportation
Q&A with Hrishikesh Tawade, Tech Lead in Robotics and Computer Vision | Ample
Tell us about yourself and your role with Ample.
I’m Hrishikesh Tawade, and I serve as the Tech Lead for Robotics and Vision at Ample Inc. I completed my undergraduate degree in Electronics and later earned my Master’s in Robotics from the University of Maryland.
My main focus is guiding our teams to build the next generation of EV battery-swapping stations—ones that operate faster, hold more battery capacity, and stay resilient even under heavy usage. I help define milestones and minimum viable products (MVPs) so we can refine our designs quickly in response to field data. My work also involves coordinating with mechanical, electrical, and software teams to incorporate new safety protocols for battery-swapping robots, develop speed optimization algorithms, build robust perception systems, and integrate station health monitoring and predictive maintenance tools.
I also enjoy mentoring my team of senior engineers and interns, ensuring that we strike the right balance between innovation and reliable engineering practices. In this role, I take pride in pushing the boundaries of automated battery swapping, making EV adoption easier and more accessible worldwide.
Your career has spanned multiple facets of robotics and computer vision. What drew you to this field initially, and how have you seen it evolve during your professional journey?
Growing up, I was always captivated by the idea of machines that could “see” and make decisions independently. My first real exposure to this field came during an internship at the Bhabha Atomic Research Center in India, where I had the opportunity to repair a radioactivity-detection robot designed for nuclear facilities. I vividly remember the moment when the four-wheeled robot finally came to life after being inactive for a long time, moving, sensing, and responding to instructions—it felt amazing.
Over the years, I’ve witnessed an enormous leap in capabilities—starting from simpler robotics platforms using basic sensor fusion to advanced AI-driven perception systems that handle 3D point clouds, interpret images in real time, and even learn from massive synthetic datasets. Deep learning frameworks, GPU-based processing, and sophisticated simulation tools have cut development cycles dramatically, letting us experiment faster and deploy solutions at scale.
From your perspective, what are the most significant challenges in developing reliable robotic systems for industrial applications, and how are engineers addressing these challenges today?
One big challenge is ensuring reliability in unpredictable, real-world environments—factories, warehouses, roads, or battery-swapping stations where lighting, temperatures, weather, and other miscellaneous interference can vary widely. Sensor inaccuracies and hardware constraints only add complexity. Another hurdle is seamless integration: robots need to coordinate with each other, with external infrastructure, and with human operators.
To address these issues, engineers are building robust error-handling mechanisms, redundant sensor setups, and sophisticated state machines that account for all sorts of failure modes. For instance, at Ample, we run hardware-in-loop tests and extensive simulations to catch issues early, then refine these systems further with real-world feedback. This mix of thorough testing, sensor fusion, redundancy, and flexible architectures helps keep industrial deployments stable and effective.
Computer vision and perception systems are critical components in modern robotics. How do you see these technologies maturing, and what recent developments do you find most promising?
Computer vision has come a long way over the past decade, evolving from early Kinect-era depth sensing around 2010 to today’s sophisticated, multi-sensor platforms. Between 2012 and 2015, breakthroughs in deep learning architectures (like AlexNet and ResNet) sparked a revolution in image classification and object detection. By 2017, transformer-based networks emerged leading to models like Google’s Vision Transformer (ViT) in 2020, that use attention mechanisms to parse complex scenes more effectively than previous convolutional approaches.
During this time, sensor technology also expanded well beyond standard RGB cameras. LiDAR solutions from companies such as Velodyne, Ouster, and (formerly) Quanergy have significantly dropped in cost while improving resolution, enabling millimeter-level accuracy in 3D mapping. Radar and infrared sensors pioneered by automotive giants like Tesla and Hyundai now offer long-range perception even in poor lighting or bad weather. Meanwhile, infrared technologies from FLIR Systems have found industrial and defense applications that demand robust thermal imaging.
On the hardware side, edge computing has advanced rapidly. In 2018, NVIDIA launched the Jetson Xavier, followed by Jetson Orin in 2022, providing GPU-accelerated AI capabilities on embedded devices. This shift allows robots to make near-real-time decisions at the source, rather than relying on cloud processing.
Additionally, digital twin technology has advanced significantly, with solutions like NVIDIA Omniverse providing real-time collaboration, high-fidelity physics simulations, and the ability to create virtual replicas of real-world environments. These comprehensive digital twins enable robotics teams to test control algorithms, optimize designs, and run thousands of “what if” scenarios before deploying updates to physical machines.
Alongside these digital twin platforms, synthetic data generation and simulation tools have matured. Companies like Parallel Domain, Unity, and Siemens (through its Xcelerator portfolio) are developing virtual test environments that help train vision models without requiring massive real-world datasets. By combining digital twins with synthetic data pipelines, engineers can more accurately model edge cases, reduce development cycles, and accelerate the validation process for autonomous and semi-autonomous systems.
Additionally, a technology worth watching is event-based (neuromorphic) vision, which many in the industry haven’t fully explored yet. These event-based sensors (pioneered by companies like Prophesee and iniVation) only record changes in brightness for each pixel, effectively operating in continuous time. This not only saves bandwidth but also allows for extremely high temporal resolution making it ideal for high-speed applications like drone racing, manufacturing inspection, and gesture recognition.
While mainstream adoption is still limited, the reduced data footprint and ultra-fast reaction times of event-based cameras could drive significant breakthroughs in the next few years. Coupled with emerging spiking neural network frameworks, these sensors might enable new kinds of intelligent systems that respond to dynamic environments much more efficiently than conventional frame-based solutions. This technology could reshape how robots and autonomous machines perceive and act in the world.
All these developments—transformer-based vision models, increasingly diverse sensor suites, and powerful edge hardware—have accelerated the adoption of autonomous and semi-autonomous systems across industries. As these technologies continue to mature, we can expect robots and AI-driven machines to operate with greater independence, accuracy, and reliability than ever before.
The EV industry is facing various hurdles in mass adoption. Based on your industry experience, how do you see robotics and automation helping to address some of these barriers?
There are several hurdles to EV adoption that can slow down mainstream acceptance. Long charging times remain a top concern—today’s fast-charging stations can still require 20 to 60 minutes, which is a big change compared to the 2 minutes on an average it takes to fill a traditional gas tank. Limited charging infrastructure remains a primary hurdle for EV adoption, especially outside urban cores. In the United States, major metro areas average roughly 65 public fast-charging stations per 1,000 square miles, while the national average beyond these hubs dips to only 18, compared to around 960 gas stations. Even where fast-charging is available, installing high-power chargers often requires costly grid upgrades; for instance, a proposed 600 kW highway fast-charge site in a rural area was estimated to cost over $3 million for a substation extension alone.
Battery swapping offers a potent alternative, as robotics-enabled systems can replace depleted packs with fully charged units in a matter of minutes, effectively matching or surpassing the convenience of refueling at a gas station. Nio’s Power Swap stations in China, for example, complete a fully automated battery exchange in five minutes or less, and are designed to handle more than 300 swaps per day. This effectively mirrors the refueling pattern drivers are accustomed to with gasoline cars, eliminating the psychological barrier of long charging downtime.
Battery swapping not only delivers near-instant “refueling” but also ties into the concept of “battery-as-a-service,” meaning users no longer purchase the battery outright. By subscribing to a monthly battery plan, Nio’s EV buyers can reduce their vehicle’s sticker price by up to 70,000 RMB (about $10,000 USD), all while avoiding long-term degradation concerns.
From an operational standpoint, the role of robotics and automation in battery swapping is pivotal. Automated guided mechanisms handle each module, reducing labor costs and minimizing human error. We at Ample have demonstrated swapping of battery modules in just five minutes using robotic platforms that slide underneath the vehicle, unlatch the depleted pack, and replace it with a charged one with minimal human oversight.
Thus, lowering the initial EV price through a service-based model, freeing drivers from range anxiety, and mitigating some of the grid upgrades required by large-scale fast charging, robotics, and automation-based battery swapping can substantially accelerate the global transition to electric mobility.
Many industries are exploring multi-robot coordination for complex tasks. What are the key considerations when designing systems where multiple robots need to work together seamlessly?
When designing systems that involve multiple robots working together, a few important considerations come into play. First, robots must communicate clearly and quickly—sharing real-time information about their current status, location, and task progress. A robust network is essential, as even slight delays in communication can lead to collisions or duplicated tasks. Secondly, each robot should receive clear instructions through centralized scheduling. I always say, “Scheduling should be centralized, while safety should be distributed as much as possible.” This way, robots can independently react to safety threats and communication delays or disruptions without waiting for central instructions, thus minimizing the risks.
Another crucial aspect is collision detection and collision avoidance. Robots must be carefully equipped with perception capabilities that cover all their blind spots. Furthermore, robots must carefully plan paths to prevent interference, relying on specialized motion-planning software to create safe trajectories. Additionally, setting specific lanes or restricted zones within the workspace can simplify navigation. Error handling is equally critical: if one robot encounters a failure or loses connection, the entire system shouldn’t halt. Instead, robots should have built-in mechanisms to adapt or reroute their tasks or bypass the problematic robot temporarily.
A Robotic FMEA (Failure Mode and Effects Analysis) is a common practice that systematically identifies potential failures within robotic systems (such as sensors, actuators, software modules, or communication interfaces), assess the severity of their impacts, evaluate how often these failures could occur, and determine how effectively these failures can be detected and addressed. This analysis helps engineers to assign a Risk Priority Number (RPN) to potential risks which should drive sensing, safety, and scheduling decisions.
Industry-specific needs also shape multi-robot systems. Warehouses prioritize efficient routes, with systems like Amazon Robotics using grid-based navigation. Manufacturing requires precise coordination, as seen in automotive production, where robots must follow strict sequences. In drone-based applications, such as drone shows or aerial inspections, coordination must manage factors like limited airspace and battery life, requiring highly dynamic task reassignment.
Lastly, a strong multi-robot system should be easy to scale up—adding new robots or replacing older ones without requiring extensive redesigns. Modular designs, standardized communication protocols, and simple interfaces ensure smooth growth and adaptability over time.
Looking ahead 5-10 years, which emerging technologies do you believe will have the most transformative impact on industrial automation and robotics?
I see AI-driven autonomy and digital twins as transformative technologies that will significantly shape the future of robotics and industrial automation. With AI-driven autonomy, robots will increasingly make sophisticated real-time decisions without constant human oversight. For example, warehouse robots might automatically reorganize inventory based on predicted demand.
Digital twins, which are virtual replicas of physical systems, can be created using platforms like NVIDIA’s Omniverse and Siemens’ Xcelerator enabling engineers to simulate and test software updates, layout adjustments, and workflow optimizations before making any real-world changes. For example, digital twins can replicate complex industrial environments, from assembly lines in automotive factories to power grids, letting teams safely experiment with different scenarios, anticipate issues, and refine their systems without costly disruptions.
Enhanced edge computing will also be pivotal. Advances like NVIDIA’s Jetson Orin and Qualcomm’s robotics-focused processors allow robots to process large amounts of sensor data instantly on-device, reducing reliance on cloud infrastructure. This drastically lowers latency, enabling robots in sensitive applications—such as healthcare or autonomous vehicles—to respond swiftly and safely.
Lastly, collaborative robotics (cobots)—where humans and robots work side-by-side—is rapidly advancing. Companies like Universal Robots and Boston Dynamics are creating robots designed explicitly for safe human interaction, opening up new possibilities in traditionally manual fields like assembly, logistics, and even healthcare. Cobots equipped with intuitive interfaces and safety features can expand automation into areas previously inaccessible due to safety concerns or complexity.
Together, these emerging technologies promise a more intuitive, flexible, and efficient future for robotics, reshaping industries in ways we’re only beginning to explore.
The content & opinions in this article are the author’s and do not necessarily represent the views of RoboticsTomorrow
Comments (0)
This post does not have any comments. Be the first to leave a comment below.
Featured Product
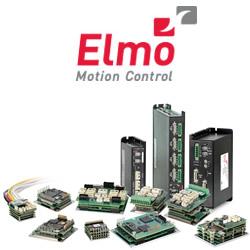
Elmo Motion Control – The Platinum Line, a new era in servo control
Significantly enhanced servo performance, higher EtherCAT networking precision, richer servo operation capabilities, more feedback options, and certified smart Functional Safety. Elmo’s industry-leading Platinum line of servo drives provides faster and more enhanced servo performance with wider bandwidth, higher resolutions, and advanced control for better results. Platinum drives offer precise EtherCAT networking, faster cycling, high synchronization, negligible jitters, and near-zero latency. They are fully synchronized to the servo loops and feature-rich feedback support, up to three feedbacks simultaneously (with two absolute encoders working simultaneously). The Platinum Line includes one of the world’s smallest Functional Safety, and FSoE-certified servo drives with unique SIL capabilities.